Big data brings new challenges to the world of analytics in terms of unstructured data types, very high volumes of data/streaming data, and events that need to be used as triggers for some analysis of that data.
What is a Data Lake?
The need to handle so much data and make sense out of it, combined with enterprise system data, led to the creation of the data lake concept. A data lake brings together data from different sources to clean the data, identify its source, ensure that it follows common business semantics for an organization, and make it accessible to the right users for further analytics, often in a self-service mode.
The data lake concept has evolved along with advances in big data concepts because big data brings new challenges to the world of analytics in terms of unstructured data types, very high volumes of data and streaming data, and events that need to be used as triggers for data analysis.
The following are some of the key motivations behind the data lake concept:
- Data needs to be analyzed, but huge amounts of data are difficult to store in high-performance and advanced analytical systems, such as SAP HANA. The storage cost would be extremely high, and it’s not important to store all the data in this way. This then requires data storage beyond data warehousing systems (such as SAP BW on SAP HANA or SAP BW/4HANA) and more cost-efficient yet easy-to-integrate systems
(such as Hadoop clusters). - An infrastructure is required that can store these analytical repositories without dependency on a specific data format. In other words, you need an infrastructure that can handle structured, unstructured, or semistructured data.
- The data lineage from the source system should be traceable. This is also useful for regulatory compliance.
- Business users need to be able to use data for self-service analytics.
- Application rationalization needs data to be in a well-architected format in a few systems, rather than all over the landscape with several different types of data warehousing solutions and visualization tools and even more custom-built solutions, which make maintenance a nightmare for IT and ease of use a nightmare for business users. A structured approach makes it possible to use the data in a publish-subscribe mode.
- The data will eventually move into the transactional systems and hence needs to be of guaranteed quality; thus, the governance framework plays an important part.
- Proper metadata management capability is important for analysts to understand the data they’re consuming and a must-have for the data governance processes and data management processes.
The figure below shows how the data lake sits in an application landscape and interacts with other systems and how users interact with it.
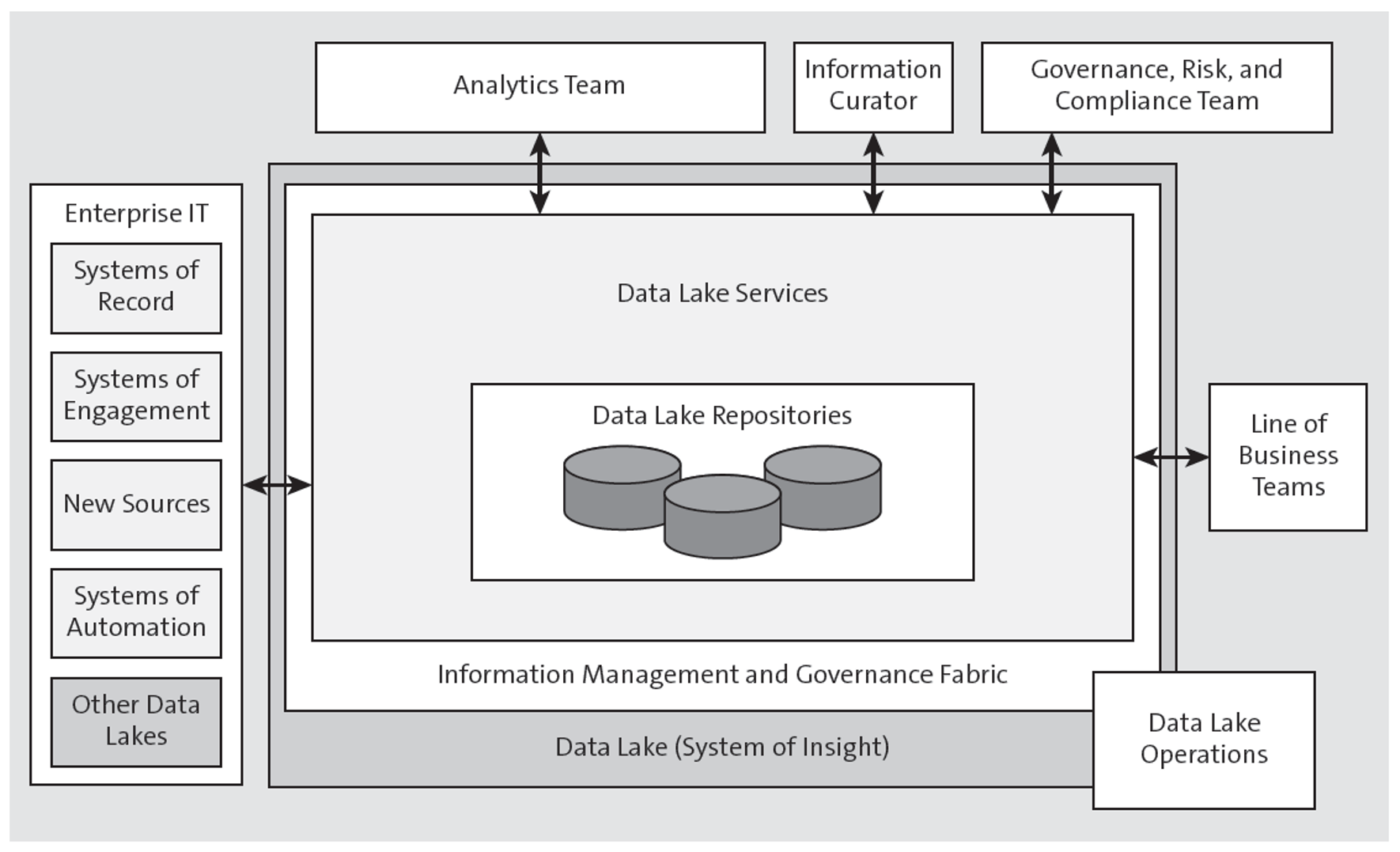
The outer edge of the figure shows the various users of the data lake:
- The analytics team is a group of users, including data scientists, that is responsible for carrying out the advanced analytics across the data lake.
- The information curator is responsible for the management of the data catalog, which users will employ to find relevant data elements in the data lake.
- The governance, risk, and compliance team is responsible for defining the overall governance program of the data lake and any associated reporting functions to demonstrate compliance.
- The data lake operators are responsible for the day-to-day operations of the data lake.
- The LoB users might have roles such as manufacturing line users, finance users, sales team, and so on.
Each organization must formulate the data management and data governance principles it wants to follow and include its desired metadata model, which should be easy to extend and have a set of semantics that is common across the business.
The data lake needs to be connected to the data sources throughout the enterprise through suitable governance—thus ensuring consistency and controllability of data.
All hyperscalers, such as AWS and Microsoft Azure, have come up with their data lake architectures and positioned their products, aimed to not only help with the storage but also with the governance and the various support functions described previously.
SAP Datasphere
One of the major challenges with data lakes is the lack of proper governance in terms of data ownership, data quality standards, data lineage, and reusability. Also, IT teams often have to massage data into consumable information, while business teams find it difficult to leverage data from the data lake to the extent required. This is because of the loss of business context when data is pulled out of the business systems. These challenges led to the advent of data fabric, which is the solution for automating data management from multiple sources of data at any point in time, streamlining data, and enriching it through cleansing it, unifying it, securing it in complex distributed architectures, and making it ready for consumption by analytics and AI.
SAP Datasphere is a solution that has capabilities in this area. It provides the right platform for leveraging business data, and it uses AI tools from SAP BTP to bring major advantages to business through AI-enabled business analytics. The blog at the following link explains well how SAP Datasphere acts like a data fabric: http://s-prs.co/v597313.
In the next few years, data fabrics will work together with data lakes to provide the best data experience. They will also provide access to self-service analytics on huge amounts of authentic and semantically aligned data from multiple sources and more functions.
Conclusion
As big data has grown more beneficial to businesses, companies such as SAP needed to provide solutions to make sense of large swaths of data. With tools such as SAP Datasphere and SAP HANA, data analysts can utilize the popular data lake format of data storage as a way to sort through big data with SAP.
Editor’s note: This post has been adapted from a section of the book SAP S/4HANA: An Introduction by Devraj Bardhan, Axel Baumgartl, Madalina Dascalescu, Mark Dudgeon, Piotr Górecki, Asidhara Lahiri, Richard Maund, Bert Meijerink, and Andrew Worsley-Tonks. They are are a multinational author team working for IBM, SAP, and Accenture. They have
been working with SAP S/4HANA since its first release.
This post was originally published 11/2019 and updated 3/2025.
Comments